Grid filtering is a useful technique in Artifical Intelligence that lets you determine the true state of your environment from a noisy sensor. As an example, imagine you were a tank in a 3D world that you had never explored. Of course, to make this contrived example less realistic, I will assume you can only “see” what is in the world through your noisy sensor, which basically tells you if there is an object near you. Thing is, by “noisy”, we mean it doesn’t always return accurate data. Instead, most of the time it tells the truth, but because it isn’t perfect it sometimes lies. Using Bayesian reasoning, we can then sample several times in one spot to pin down the true state. Where one single sample might leave us uncertain, more than say 5 or 10 samples should leave us almost certain (but we’re never really 100% sure, just as I feel in my dating life).
So we implemented this grid filter based of some code that Dr. Goodrich provides. We then produce an occupancy grid where black represents “likely” objects and white represents nothing. We then take it a step further to find corners, and from these corners infer boxes. Below are a few images that show the “believed” state of the world after scanning through it using our sensor and the grid filter logic. On top of this, we have overlaid translucent red or blue squares to indicate obstacles. Youll also notice tick marks around these squares, which are simply to indicate where our corner finding algorithm thought the obstacle edges were.
The cool thing is, this is really simple and useful, since just about any sensor exhibits some noise and hence uncertainty in its interpretation. This particular example works best for static worlds. The Kalman filter is better at modeling dynamic things, seems.
Below: Map of our “world” after using a grid filter and corner detection. Note how “noisy” this is, and yet we were still able to find all our obstacles (the dark black lines). Also note that the colored squares, which are our “believed” obstacles, sometimes cover regions that are larger than what our true obstacle are. For example, around the “L” shaped obstacles, we have a simple square. This works, because we don’t want to get stuck in an “L” anywise (our code for getting our tank out of concave regions isn’t, well, there, really)
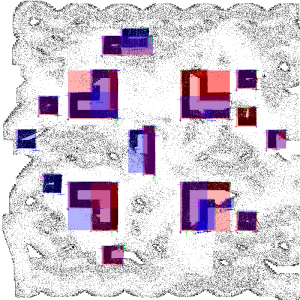